Modeling deception
Text block one
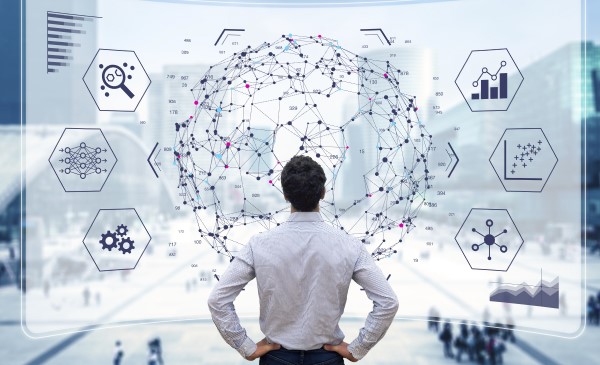
When two people communicate, it changes the knowledge of both the listener and the speaker, and potentially enhances mutual beliefs.
But how do people know what they are hearing, or what is being communicated to them, is true? Often they weigh factors such as prior knowledge and the physical actions of the speaker. Researchers are now examining how that same thinking can also apply to artificial intelligence (AI) models.
Associate Professor Piotr Gmytrasiewicz is training AI systems to account for and model deception, allowing a particular system to weigh not only a message it got from another AI-enabled device, but also its own observations, physical actions (such as the movements of an AI-enabled robot), and messages it previously sent to determine what is true.
He uses Bayesian decision theory — a mathematical procedure that applies probabilities to statistical problems — which allows AI tools to update their beliefs when they receive evidence of new data. It combines prior information with what it already knows about the speaker’s sincerity to evaluate communicative acts.
Sometimes an AI model cannot directly observe what is happening, so the system needs a way to counter the ambiguity in the model to build a clearer picture of probable outcomes. Gmytrasiewicz uses interactive POMDPs (partially observable Markov decision process), another mathematical framework, to reinforce learning.
Gymtrasiewicz’s approach to communication does not make the common assumption that systems are cooperative. He is enabling AI systems to plan for deception and guard from the deceptive behaviors of others.